Overview
Predictive maintenance (PdM) methods actively monitor process and equipment parameters to determine optimal timing for maintenance. Control charts are used to monitor performance, trigger maintenance activity and improve Overall Equipment Effectiveness (OEE) for more productive manufacturing systems. Using process based analytics enables event-driven maintenance which produces better product at lower cost with less downtime.
PdM is the strategy of choice to be compliant with modern manufacturing operations management standards such as ISA-95. Manufacturing Intelligence (MI) provides the real-time role-specific reporting of process analytics that enables coordinating maintenance operations management with optimized production operations.
Maintenance Models
The frequency and timing of maintenance activities is an exercise in balancing cost and risk. Maintenance delayed too long leads to catastrophic failure with high costs for repair or replacement. If maintenance is more frequent than needed, the cost, which includes downtime, opportunity costs, and the cost of consumables, parts, and labor, will be greater than needed to provide dependable operation.
Conventional maintenance programs operate under fixed schedules. Since these programs do not directly monitor the state of the process, scheduled maintenance has to be excessively conservative to prevent failure. Generally there is no value-add to this large safety margin with its associated cost and overhead.
The real value-add strategy is predictive maintenance (PdM), also known as condition monitoring, which applies statistical process control (SPC) methods to detect and eliminate equipment problems that can lead to unplanned downtime and unnecessary expenditures.
Once MI is in place to monitor the state of the process and predict process degradation, the company can balance cost and risk and be both more efficient and cost effective. Maintenance becomes event-driven, triggered by SPC signals and managed by lean just-in-time methods.
This approach fits into modern manufacturing operations management (MOM) models using MI as the enabling technology. This manufacturing strategy depends on real-time, role-specific reporting of process analytics to support process management decision making.
The analytics enable operators to detect process destabilization and changes in the process capability to meet specifications. Scheduled maintenance systems typically have no means to be aware of process disturbances. With continuous monitoring and process analysis, operations can move to an appropriate “just-in-time” event-driven maintenance response.
Process analytics are the most effective method to drive event-driven maintenance because they:
- Are applicable to monitoring any parameter whether measuring pathogen levels, shaft load, bearing wear, etc.;
- Differentiate signals from noise;
- Are more sensitive to equipment and process deterioration than simple critical value based inspection systems;
- Enable dynamic, “intelligent” system response.
The core analytics used to monitor the process and trigger maintenance activities are control charts and process capability analysis.
Control Chart
The control chart (figure 1) monitors process stability and give signals when the charted parameter shows unpredicted process drift. The ability to monitor pattern rule violations provides greater sensitivity to process degradation than simple violations of critical values.
Process Capability Report
Process capability analysis (figure 2) examines and quantifies the ability of a particular process to perform to specification. Process capability gives a reading of process variation. By using this information, staff can determine the likelihood of reaching a given value and set the risk level to trigger maintenance.
ISA -95 Analytics and Predictive Maintenance
PdM needs to operate within a structured MOM environment where the relationships between each operation are defined along with data and command transfer. The ISA-95 standard defines MOM activity models which provide a structure for a manufacturing data system. ISA-95 is the result of ongoing collaborative effort by major systems vendors and corporate users working within the International Society for Automation (ISA). The committee has mapped the data sources within a manufacturing enterprise and the processes that form operations and management. Each level considers:
- Industry best practices;
- How processes operate;
- The data required to monitor each process;
- How the data is analyzed to manage that process;
- Reporting required by those operating that process and those managing it;
- Information shared between different functional departments within the company and how it is meaningfully analyzed and reported;
- How data from different sources is aggregated to produce Key Performance Indicators (KPIs) which integrate the manufacturing and business management concerns of the company.
This model enables combining business and manufacturing data to better support enterprise management and decision making. One can drill down in each level in the model for the detailed data structures and workflows in sections of the business. For example, the sections and interactions for Level 3, Manufacturing Operations Management, are described in figure 3.
The MOM domain is defined to include production, quality, maintenance and inventory operations management functions, tasks within functions, and exchanges between tasks in an operations sequence. The following are the four fundamental MOM activities:
Production Operations Management: Management activities within Level 3 of a manufacturing facility which coordinate, direct, manage and track the functions at each operation that consumes raw materials, energy, equipment, personnel and information to produce products to the required costs, qualities, quantities, safety and timeliness. MES functions are mostly derived from this activity model with a lesser number from the supporting activity models below.
Maintenance Operations Management:Management activities coordinate, direct and track the functions that maintain the equipment, tools and related assets to ensure their availability for production operations. This includes changeover, setup and clean-in-place activities and operations.
Quality Operations Management: Management activities coordinate, direct and track the functions that measure and report on quality test activities in support of production operations. The broad scope of quality operations management includes both quality operations and the management of those operations to ensure the quality of intermediate and final products.
Inventory Operations Management: The activities coordinate, direct and track the functions that transfer materials between and within work centers and manage information about material locations and statuses in support of production operations.
For a more detailed explanation of the above models or the ISA-95 standard, refer to ANSI/ISA–95.00.03–2011 - Enterprise-Control System Integration Part 3: Activity Models of Manufacturing Operations Management.
The Maintenance Operations Management section of ISA-95 is shown in Figure 4. As for all four activities, process monitoring data collection and decision support analytics are core parts of the process definition. MI is the method to provide these enabling functions and PdM is the maintenance strategy that fits within the intent of ISA-95.
This systems view of maintenance operations within the MOM model is designed to be applicable to any manufacturing company regardless of the industry. Examples of systemic applications of PdM in two different industries are below.
Process Analytics Enable Food Safety and Predictive Maintenance
NWA participated in a study at Butterball Turkey which demonstrated that process management using SPC methods was the effective way to make a critical value based HACCP inspection system work. In the Butterball case, aggressive use of SPC methodology enabled the staff to detect performance failure in the wash cabinet and to make timely maintenance and process adjustments.
Because trained people were monitoring control chart signals, they were able to identify and to deal with inadequate wash cabinet performance and avoid shipping unwholesome food. The Butterball case illustrates how using analytics to monitor critical process variables enables process operators and management to sense process state and respond in timely fashion to prevent system failure.
While the study focused on how process management methods enable successful HACCP systems, it also demonstrated how well analytics perform in triggering maintenance activities. By understanding underlying process behavior, maintenance can be performed on the most cost-effective, least disruptive schedule.
e-Diagnostics – PdM for Semiconductor Manufacturing
Advances made by one industry provide effective lessons and models for other industrial processes. Semiconductor manufacturing requires aggressive maintenance to maintain productivity and profitability. The industry cannot afford the cost inefficiencies of scheduled maintenance and has spent considerable effort to develop predictive methods that will trigger just-in-time maintenance activities.
International SEMATECH (ISMT) is a global consortium focused on advancing semiconductor manufacturing technologies. The ISMT Manufacturing Methods and Productivity (MM&P) Division has defined e-Diagnostics as an advanced monitoring, analysis, and reporting model for PdM. While not all manufacturing systems will require such sophisticated maintenance management, it is an excellent reference model and source of good ideas.
The ISMT e-Diagnostics program brings together IC makers, equipment suppliers, and software suppliers to define requirements to leverage mainstream computing technology, including the Internet, to improve OEE, drive down equipment support costs, reduce the mean time to repair, and consequently to increase output through higher availability.
e-Diagnostics operates in a process management context (figure 5). SPC and associated process analytics are an integral part of Level 2 - the analysis part of the system.
The e-Diagnostic system provides both historical and real-time information and uses control charts and other visualization methods to present it in a meaningful and user-accessible manner. Active use of analytics improves productivity and the OEE of factory operations as shown in the white paper, Applying Manufacturing Intelligence to OEE for Real-Time Decision Support.
Summary
Event-driven predictive maintenance programs guarantee equipment availability and performance. They become a core component of successful manufacturing operations management. PdM supports manufacturing excellence by:
- Operating in active problem prevention mode. Typical scheduled systems are passive and reactive. By taking an active approach, operations can make maintenance part of the continuous improvement program and reduce the likelihood of a system failure.
- Active deployment of automated data collection systems to improve data quality and reduce operator overhead.
- Using process analysis to increase system knowledge and develop appropriate responses to prevent process failures.
- Monitoring process performance to drive optimized, event-driven maintenance frequency for greater cost-effectiveness and performance.
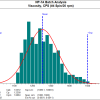